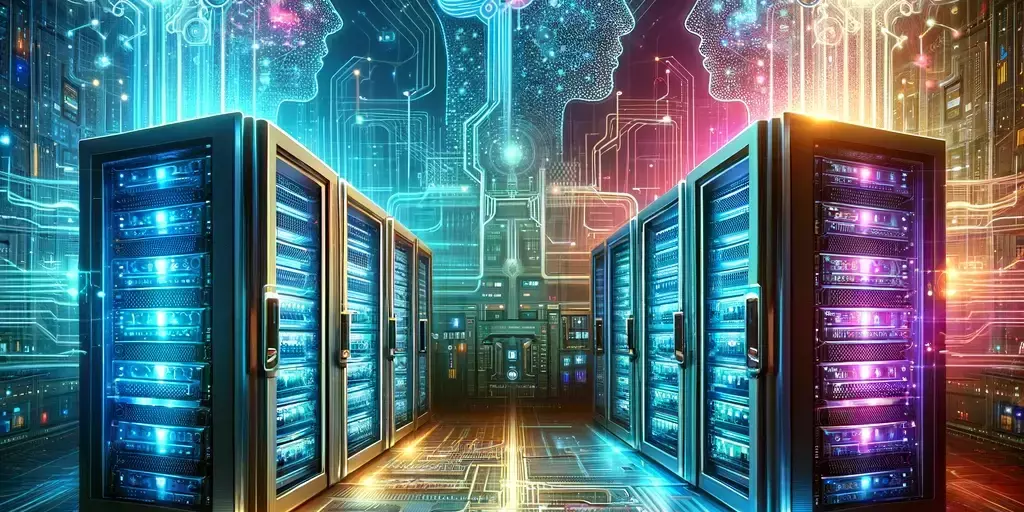
Generative AI, a subset of artificial intelligence, excels in creating new and original content by learning from existing data. But not all data is equally suitable for these models. Understanding which types of data are most compatible with Generative AI can significantly enhance its applications and outputs. This blog explores the various data types ideal for Generative AI and offers insights into optimizing their use.
Types of Data Suitable for Generative AI
1. Text Data: Generative AI shines in processing and generating text. This includes writing articles, creating code snippets, and generating creative content like stories or poetry. Models like GPT‑3 and GPT‑4 analyze language patterns to produce coherent and contextually relevant text.
Applications:
- Automating content creation for blogs and articles.
- Assisting in writing marketing materials.
- Generating programming code and scripts.
2. Image Data: Generative AI can create realistic images, thanks to models like Generative Adversarial Networks (GANs). These models learn from vast datasets of images to produce high-quality visuals that mimic real photos.
Applications:
- Designing new and innovative visual elements.
- Creating unique patterns and designs in fashion and graphic design.
- Generating synthetic images for training machine learning models.
3. Time-Series Data: For applications that require understanding temporal patterns, such as stock market predictions or weather forecasting, Generative AI can analyze time-series data to make accurate predictions.
Applications:
- Financial market analysis and prediction.
- Weather forecasting and climate modeling.
- Monitoring and predicting system behaviors in IoT applications.
4. Multimodal Data: Combining text, images, audio, and other data types, multimodal Generative AI models can understand and generate comprehensive outputs that span multiple modalities.
Applications:
- Enhancing social media content with integrated text and visual elements.
- Creating interactive and engaging multimedia experiences.
- Analyzing and generating rich datasets that include multiple data types.
5. Structured Data: Generative AI can handle structured data from databases and spreadsheets, generating new entries or predicting missing values. This is particularly useful for augmenting datasets and improving data quality.
Applications:
- Synthesizing new data to fill gaps in existing datasets.
- Enhancing machine learning model training with additional synthetic data.
- Automating data entry and correction tasks.
Optimizing Generative AI for Different Data Types
1. Training Data Quality: The performance of Generative AI models heavily depends on the quality of the training data. Diverse, representative, and unbiased datasets lead to better model outputs.
2. Model Architecture: Selecting the appropriate model architecture is crucial. For instance, recurrent neural networks (RNNs) are suitable for sequential data, while convolutional neural networks (CNNs) excel with image data.
3. Hyperparameter Tuning: Fine-tuning hyperparameters such as learning rate, batch size, and regularization techniques can significantly enhance model performance and generative capabilities.
Conclusion
Generative AI is a powerful tool that can revolutionize content creation, data analysis, and predictive modeling. By understanding the types of data most suitable for Generative AI and optimizing model training and deployment, businesses can harness its full potential. From text and images to time-series and structured data, the right data types can drive innovation and efficiency across various domains