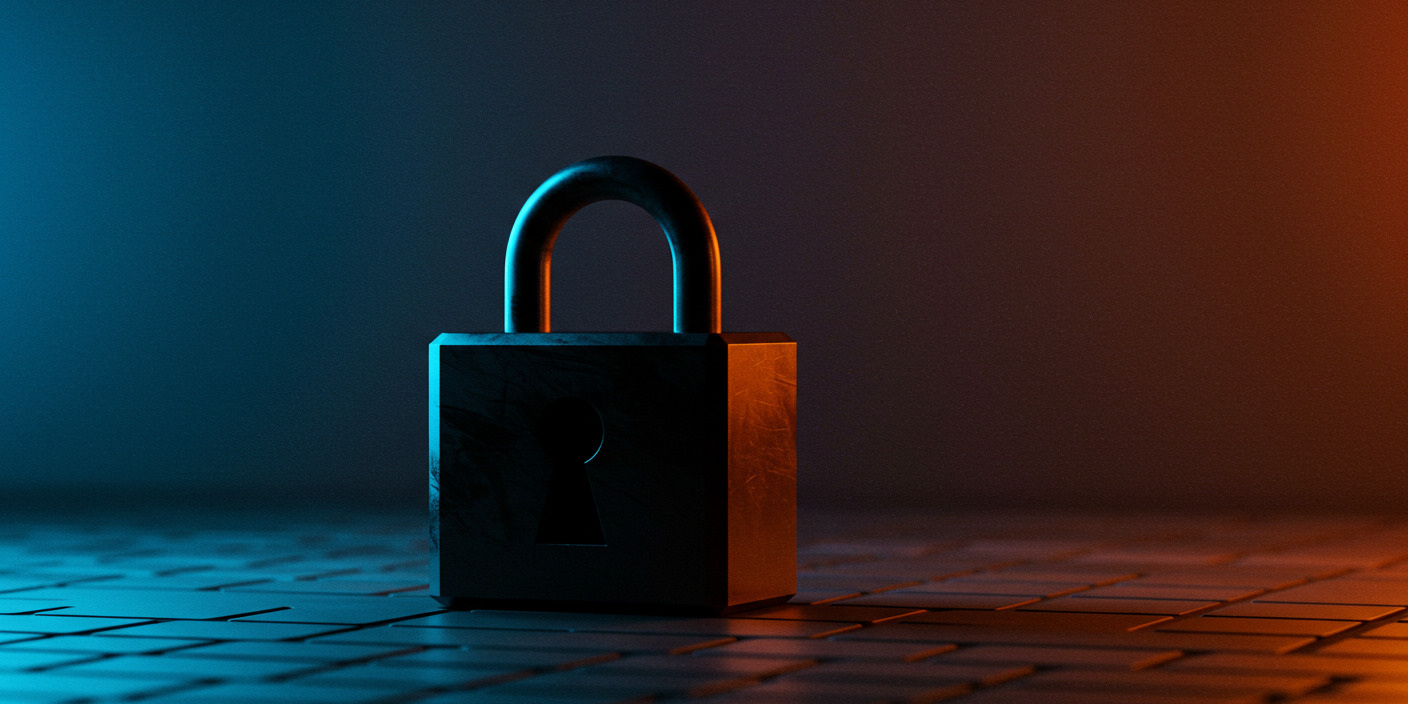
The conversation around AI used to be all about power — how fast, how smart, how scalable. But now that generative AI models are being deployed in everything from customer service to credit decisions, we’re facing a new kind of question: Can we trust it?
That’s where transparency and accountability come in. They’re not just abstract ethics buzzwords. They’re the backbone of responsible AI deployment — especially as generative models become more integrated into everyday business operations. And yet, most organizations are still figuring out what those words mean in practice.
Let’s talk about why transparency and accountability are hard to get right, what’s at stake when we get them wrong, and how a few companies are beginning to show the way forward.
What Does AI Transparency Actually Mean?
Transparency, in this context, doesn’t just mean open-source code or a peek into model weights. It’s about understandability. How was the model trained? What data was used? How are outputs generated, and what assumptions are baked into those outputs?
And maybe most importantly: if the AI gets something wrong—who is responsible?
Take OpenAI’s ChatGPT. It’s astonishingly useful, but also famously opaque. As of early 2024, we still don’t know the full scope of the data used to train it. That makes it incredibly difficult to audit for bias, misinformation, or IP infringement. And yet, it’s being used in everything from law firms to schools.
If a law firm uses ChatGPT to draft a legal brief and it fabricates case law (which has happened), who’s accountable? The user? The developer? The AI?
Without transparency, those questions get harder — not easier.
Why Accountability Isn’t Optional Anymore
Accountability goes hand in hand with transparency. It’s about ownership of outcomes. When a model discriminates against a job applicant or denies someone a loan unfairly, accountability means someone answers for that decision — and fixes it.
Consider the case of Apple Card. In 2019, users began reporting that the Apple-Goldman Sachs credit card algorithm was offering dramatically different credit limits to men and women with similar profiles. The company claimed that no gender data was used in decision-making. But that’s the thing about machine learning — bias can leak in through proxies.
Goldman eventually admitted the algorithm had issues, and regulators launched an investigation. The real problem? The model was a black box, even to those deploying it. There was no clear chain of accountability.
That’s not just a PR issue. It’s a governance failure.
What Makes AI Governance So Difficult?
Unlike traditional software, AI systems evolve. They learn, they retrain, and they sometimes behave in unexpected ways. That makes it harder to apply standard risk or compliance frameworks.
A few key challenges:
-
Opacity by design: Many AI models (especially deep learning models) are inherently difficult to interpret. You can’t easily trace a specific output to a specific input.
-
Third-party dependencies: Organizations often don’t build their own models. They integrate APIs from OpenAI, Google, Anthropic, and others — adding layers of distance between use and responsibility.
-
Dynamic data: If a model updates in real time based on new data (say, customer sentiment or user clicks), governance isn’t a one-time effort. It’s an ongoing process.
This complexity doesn’t mean we give up on transparency. It means we rethink what transparency looks like in an AI-powered environment.
What Good Governance Looks Like (and Who’s Doing It Well)
The organizations getting this right are the ones treating governance as a design constraint, not an afterthought.
-
Microsoft has been building out responsible AI documentation toolkits, including model cards and system datasheets that explain what models are, how they should be used, and what risks they carry.
-
Mozilla, through its Mozilla.ai initiative, is pushing for “trustworthy AI” that includes auditable documentation, bias evaluations, and community feedback baked into development cycles.
-
Airbnb established a cross-functional AI ethics review board to evaluate sensitive deployments — like how AI is used in fraud detection or guest screening.
These aren’t just checkboxes. They’re active, living processes that embed transparency and accountability into the culture of how AI is used.
So, Is This Really Game-Changing?
In short: yes, if we treat it that way.
We’re entering a phase where AI doesn’t just support decisions — it makes them. That kind of power, in the absence of visibility or responsibility, is dangerous. But with the right governance, it’s a massive opportunity. It means companies can build trust with users. It means regulators can better understand emerging tech. It means AI can scale safely.
Will it slow things down? Sure. But maybe that’s a good thing.
We don’t need AI to move faster. We need it to move smarter. Governance is how we get there.
Final Thoughts: Don’t Build in the Dark
Transparency and accountability aren’t just about ethics — they’re about risk management, brand reputation, and long-term sustainability.
You don’t need to open-source your entire model. But you do need to be able to explain how it works, monitor what it’s doing, and own the consequences when it misfires.
Because in the end, trust isn’t built on what your model can do. It’s built on how clearly you can explain why it did it.