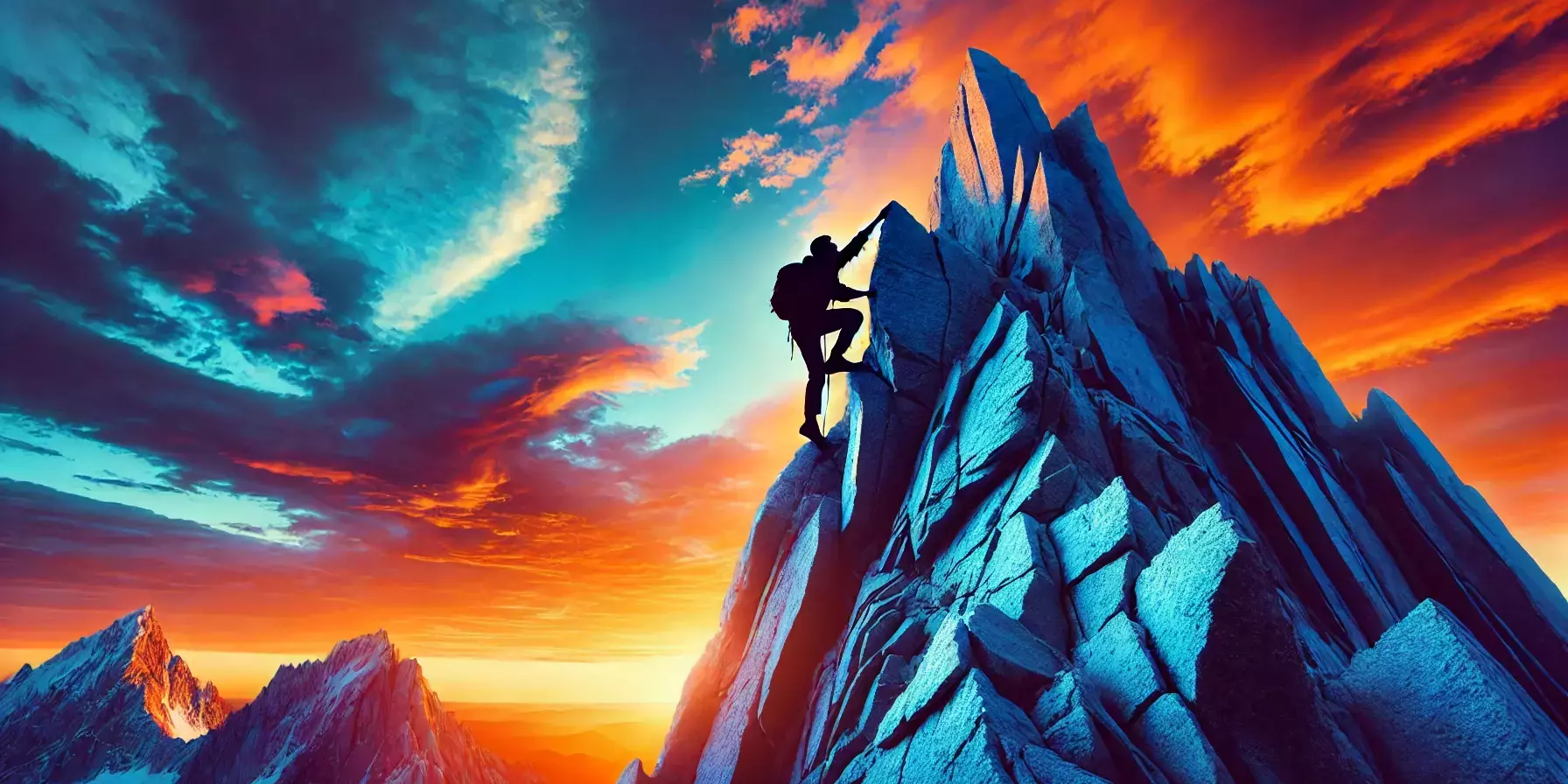
AI-driven business transformation is an exciting journey for innovators. It offers the potential to revolutionize industries, streamline operations, and enhance customer experiences. However, the path to successful AI adoption is not without challenges. Businesses must navigate technical, cultural, and operational obstacles to fully realize AI’s transformative power. In this article, we’ll explore some of the most common challenges in AI-driven business transformation and provide actionable strategies to overcome them.
1. Data Quality and Integration Issues
AI thrives on high-quality data, but many organizations struggle with fragmented, unstructured, or inconsistent data. AI models need clean, reliable, and well-organized data to generate accurate insights and predictions. However, businesses often face data silos, where valuable information is stored in disparate systems, making it difficult to access or use effectively.
Challenges:
- Fragmented Data Silos: Data scattered across various departments or platforms hinders AI’s ability to provide a holistic view.
- Unstructured Data: Many businesses have a large amount of unstructured data (e.g., emails, social media interactions), which can be challenging for AI systems to process.
Solutions:
- Data Integration Tools: Implementing robust data integration solutions, such as ETL (Extract, Transform, Load) tools, can help bring data from different sources into a unified system. Platforms like Snowflake specialize in integrating and cleansing data from multiple sources.
- Data Governance: Establish a clear data governance framework to ensure data consistency, quality, and security. This includes creating standards for data input, defining ownership of data assets, and ensuring regular data audits.
2. Cultural Resistance to Change
AI-driven transformation often involves significant changes in how employees work. This can lead to resistance, especially among teams who may fear job displacement or struggle to adapt to new technology. Without buy-in from employees, AI initiatives may face internal roadblocks, hindering successful adoption.
Challenges:
- Fear of Job Loss: Employees may fear that AI will replace their roles, leading to anxiety and resistance.
- Lack of Digital Skills: Many employees may lack the necessary skills to work effectively alongside AI-driven tools and systems.
Solutions:
- Transparent Communication: It’s essential to communicate the goals and benefits of AI clearly to the workforce. Emphasize how AI is designed to augment human capabilities, not replace them. Share examples of how AI can help employees focus on more strategic, creative tasks.
- Up-skilling and Training Programs: Invest in training programs to help employees develop the skills they need to work with AI technologies. Many companies are offering AI literacy workshops and creating AI centers of excellence to facilitate learning.
Example: PwC has launched AI upskilling programs for its workforce, ensuring that employees understand how to use AI in their daily tasks rather than fearing its impact
3. Lack of Clear AI Strategy
Many organizations adopt AI without a clear, overarching strategy. This results in disconnected pilot projects that fail to deliver significant value. Without a well-defined vision and roadmap, AI initiatives can become siloed and fail to scale across the organization.
Challenges:
- Isolated AI Pilots: Some businesses focus on limited-use cases without considering how AI can scale across multiple functions or departments.
- Unclear ROI: It can be difficult to measure the impact of AI initiatives, leading to uncertainty around the business value of AI projects.
Solutions:
- Define a Long-Term AI Strategy: Align your AI initiatives with your broader business goals. A clear AI strategy should include use cases that span departments, focus on high-impact areas, and align with the company’s long-term vision.
- Measure Success with KPIs: Establish key performance indicators (KPIs) for each AI initiative to track progress and measure ROI. These KPIs could include cost savings, increased efficiency, or customer satisfaction improvements.
Example: JPMorgan Chase successfully rolled out AI across its operations by first identifying high-value areas such as contract review in its legal department and then scaling AI initiatives from there.
4. Scalability Issues
Many companies successfully deploy AI in pilot programs but struggle when scaling AI solutions to the enterprise level. The complexity of scaling AI across different teams and functions can result in inefficiencies and technical bottlenecks.
Challenges:
- Infrastructure Limitations: AI models require significant computational power and data storage, which may exceed the capabilities of existing IT infrastructure.
- Technical Debt: Organizations that implement AI solutions without adequate planning may face technical debt as their systems become increasingly complex to maintain.
Solutions:
- Cloud Computing: Leveraging cloud-based AI platforms such as AWS, Google Cloud AI, or Microsoft Azure can provide the necessary computational power and flexibility to scale AI initiatives without overhauling existing infrastructure.
- Iterative Deployment: Instead of trying to scale AI across the entire organization at once, adopt an iterative approach. Start by expanding AI initiatives within one department and gradually roll out solutions across the organization as you refine processes and infrastructure.
5. Ethical and Regulatory Concerns
As businesses adopt AI, they must also navigate the ethical challenges of AI, including issues of bias, transparency, and compliance with regulatory frameworks. If not managed properly, these concerns can create legal and repetitional risks.
Challenges:
- Bias in AI Models: AI systems may unintentionally learn biases from training data, leading to unfair outcomes, particularly in areas like hiring, lending, or customer service.
- Lack of Transparency: Many AI systems function as “black boxes,” making it difficult to understand how decisions are made.
Solutions:
- Ethical AI Frameworks: Implement ethical guidelines for AI development and usage, ensuring fairness, accountability, and transparency. These frameworks should cover how data is collected, how models are trained, and how decisions are explained to end-users.
- Explainable AI (XAI): Invest in explainable AI solutions that allow users to understand how AI models arrive at decisions. This can help improve trust in AI systems and ensure compliance with regulations like GDPR or CCPA.
Conclusion: Turning Challenges into Opportunities
AI-driven business transformation is an exciting yet challenging journey for innovators. While the road to successful AI adoption can be fraught with obstacles like data quality issues, cultural resistance, and scalability challenges, these barriers can be overcome with the right strategies. By addressing these common challenges head-on, businesses can unlock the full potential of AI and position themselves as leaders in the digital age. The future of AI is bright, and for those who embrace it with a well-defined strategy and a commitment to continuous improvement, the rewards are immense.