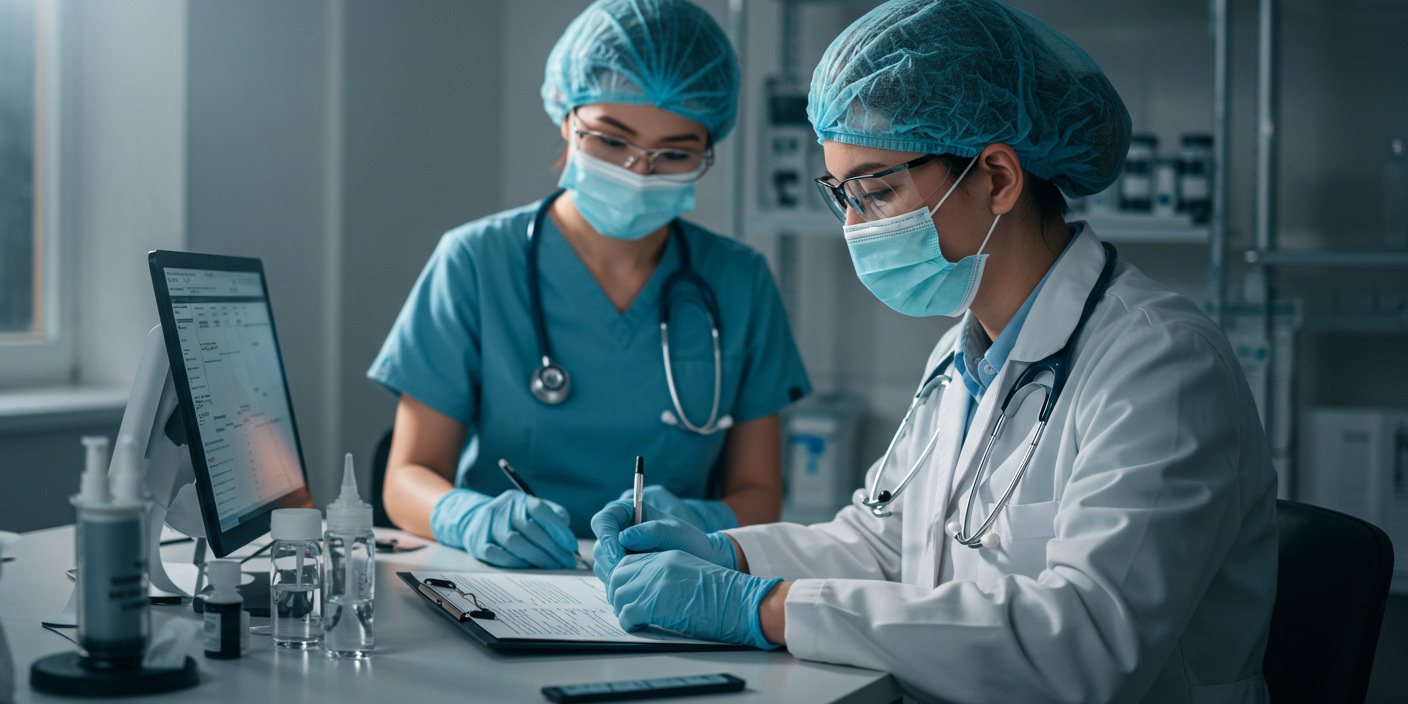
For decades, clinical trials have been the slowest, most expensive step in getting life-saving treatments to patients. Recruiting the right people, collecting the right data, managing the flood of paperwork — it all adds up to years of delay and millions in cost.
But that’s changing. AI is starting to pull clinical trials into the 21st century. Not by replacing scientists or clinicians, but by giving them sharper tools, faster feedback loops, and a clearer picture of what’s happening in real time.
If you’re in healthcare or life sciences and you’re exploring what AI can do today, this one’s for you.
Why Clinical Trials Are So Hard to Run
Before we talk solutions, let’s be honest about the problem.
Most clinical trials fail. Either they don’t recruit enough participants, don’t retain them, or the data doesn’t hold up under regulatory scrutiny. Even when they do succeed, it takes 6 – 10 years and often costs over a billion dollars to get from molecule to market.
A few sticking points:
-
Recruitment: Finding patients who meet complex eligibility criteria is like searching for a needle in a haystack.
-
Diversity: Many trials struggle to enroll participants from varied backgrounds, which affects the science and the ethics.
-
Data management: So much of the work is still manual — reviewing records, entering data, resolving discrepancies.
-
Protocol design: The trial design might look great on paper, but it doesn’t always match real-world constraints.
This is where AI isn’t just helpful — it’s a game changer.
Where AI Is Making Clinical Trials Smarter
1. Faster, Smarter Patient Matching
AI systems can now comb through electronic health records (EHRs), lab reports, and genetic data to identify eligible patients at scale.
Instead of sending out flyers or relying on word-of-mouth at a handful of hospitals, sponsors can now:
-
Use natural language processing (NLP) to scan unstructured notes in medical records.
-
Match patients to trials based on live data, not outdated registries.
-
Predict which patients are likely to drop out — and adjust recruitment accordingly.
This doesn’t just help trials fill faster. It also means fewer protocol amendments down the line.
2. Designing Better Protocols from the Start
AI models trained on years of trial data can now spot patterns humans often miss. That might mean surfacing inclusion criteria that tend to delay enrollment, or flagging endpoints that are hard to measure consistently.
In practice, this leads to:
-
Simpler protocols that are easier for both patients and researchers to follow.
-
Fewer amendments and reworks, which are among the biggest hidden costs in drug development.
-
More realistic expectations around timelines and outcomes.
Think of it like having a second brain that’s read every trial report in history and never forgets.
3. Monitoring Trials in Real Time
Clinical trials generate mountains of data — vitals, side effects, lab values, adherence logs. Managing that volume manually is slow and error-prone.
AI tools can:
-
Detect anomalies in patient data that may signal adverse events or noncompliance.
-
Automate safety monitoring and flag risks early.
-
Keep trial managers updated in near real-time, rather than waiting for monthly reports.
Instead of reacting weeks later, you’re responding to issues within hours or days.
Yes, It’s Still Early — but It’s Working
This isn’t vaporware. Companies are already seeing results.
-
A mid-sized biotech running oncology trials used AI to identify overlooked patient populations in rural hospitals. Recruitment jumped by 30%, and dropout rates fell significantly.
-
Another sponsor cut their protocol development time in half using AI-powered trial design tools that suggested more feasible endpoints.
-
A global CRO layered AI into their safety monitoring workflows and reduced the average time to detect serious adverse events by 40%.
These aren’t moonshots. They’re signs that AI is finally showing up where the work gets done.
How to Start If You’re Just Getting into This
You don’t need to rip out your tech stack or hire a full machine learning team to start using AI in trials. In fact, the most effective teams tend to take a narrow approach first.
Here’s what that looks like:
Focus on One Problem
Maybe recruitment is the bottleneck. Maybe you’re spending too much on monitoring. Pick one friction point and look for tools — or partners — that target it specifically.
Use the Data You Already Have
Most health orgs sit on years of structured and unstructured data. That’s gold for training models. The key is to clean it up and make it accessible to the right systems.
Loop in Regulatory Early
FDA and EMA are warming up to AI — but you need to show your work. If an AI model is guiding patient selection, for instance, be prepared to explain how it works, where the data came from, and what bias mitigation steps you’ve taken.
Final Thought: Clinical Trials Don’t Have to Be This Hard
The pace of drug development has always been gated by the complexity of clinical trials. That doesn’t have to stay true.
AI is giving life sciences teams a way to speed up, scale down, and get closer to the patient — all without compromising safety or rigor. We’re moving from theoretical pilot projects to real deployments that save time and money, yes — but also lives.
You don’t need a moonshot to get started. You just need a question and the right tools to explore it.
If you’re running trials and wondering how to make them faster, fairer, and less painful, AI might already be the answer.