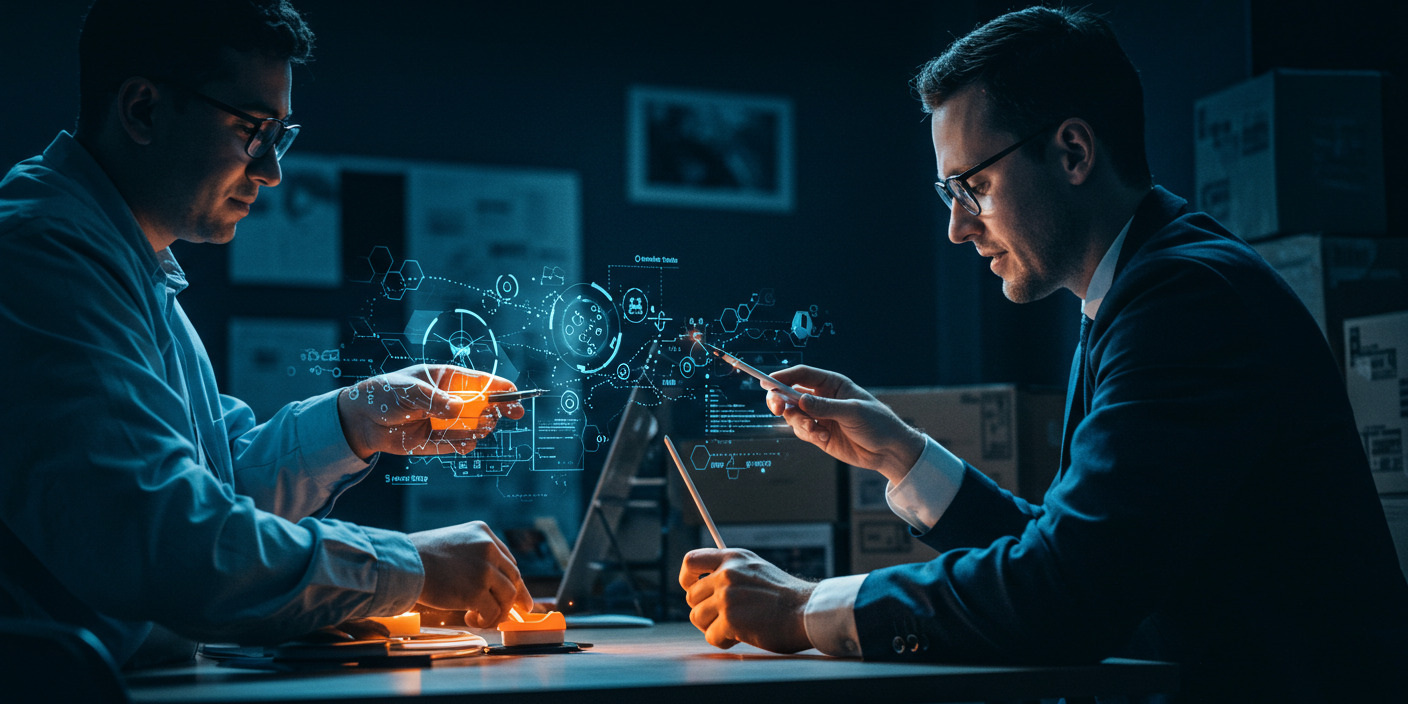
Supply chains don’t like surprises. But if the past few years have proven anything, it’s that volatility is no longer the exception — it’s the norm. From semiconductor shortages to shipping container traffic jams, businesses have learned the hard way that brittle planning systems break fast when the environment shifts.
So, what’s the alternative?
More and more companies are turning to AI — not as a magic fix, but as a way to make supply chains more flexible, more predictive, and above all, more resilient. Because in today’s world, it’s not just about forecasting. It’s about adjusting — constantly — and doing it faster than your competitors.
Let’s break down how AI actually enables this, where it’s already making a difference, and why it’s a game-changer for supply chain planning in volatile markets.
From “Best Guess” to Continuous Adaptation
Traditional supply chain planning is, at its core, deterministic. You collect last year’s sales data, maybe factor in a few seasonal trends, and build a forecast you hope holds up. But when a storm reroutes your shipping lane, or a TikTok trend triples demand for one product overnight, those forecasts fall apart.
AI flips that script. Instead of relying solely on historical data, machine learning models continuously ingest and learn from new information—sales trends, weather patterns, market signals, even social sentiment.
Case in point: During the early stages of the COVID-19 pandemic, companies using AI-driven planning platforms like Llamasoft (now part of Coupa) were able to simulate disruption scenarios and quickly adjust production and inventory levels. One global CPG company reduced stockouts by 60% compared to peers still using static planning tools.
Forecast. Adjust. Repeat.
Here’s how the AI loop works in practice:
-
Forecast: The model pulls from internal (ERP, CRM, POS) and external (market, weather, news) sources to predict demand or supply fluctuations.
-
Adjust: Based on incoming data — say, a supplier delays a shipment or demand spikes in a region — AI recommends reorders, reroutes, or inventory reallocations in near real-time.
-
Repeat: The model learns from the outcomes of those decisions, gets smarter, and continuously refines its predictions.
This cycle isn’t just more accurate. It’s more responsive. That’s what matters in a supply chain where the only constant is change.
Who’s Getting It Right?
Some standout examples:
-
Walmart uses AI to forecast demand across thousands of SKUs and stores, analyzing 200+ variables (weather, pricing, events, etc.). The result? Increased product availability and reduced waste without overstocking.
-
PepsiCo has implemented AI-powered planning to streamline distribution and production, shaving days off planning cycles and reducing excess inventory.
-
Zara, known for its fast fashion model, uses AI to optimize inventory allocation across its global store network. The company’s AI analyzes sales patterns in real time to restock high-demand items quickly, minimizing lost sales and markdowns.
These companies aren’t just using AI to forecast. They’re building supply chains that flex, learn, and evolve.
But Let’s Be Honest — It’s Not Easy
The promise of AI-driven planning sounds great, but the path there isn’t frictionless.
-
Data readiness is still a huge barrier. AI thrives on high-quality, structured data — but most supply chain data is messy, siloed, or incomplete.
-
Integration with existing systems can be painful. Many ERP and WMS platforms aren’t built for real-time AI feedback loops.
-
Trust is a cultural hurdle. Planners used to making decisions by gut instinct might be hesitant to hand control to a black box.
And then there’s the ethical side. If an AI model prioritizes suppliers based purely on data, could it unknowingly reinforce inequalities? What happens when it recommends aggressive reorders that put smaller vendors at risk? These are questions governance teams must be prepared to ask.
Transparency — into how models are built, what data they use, and how decisions are made — isn’t optional. It’s the foundation of responsible deployment.
So Is This Trend Worth the Hype?
In a word, yes.
AI in supply chain planning isn’t a passing trend. It’s a fundamental shift in how companies think about agility, resilience, and long-term competitiveness. That doesn’t mean everyone needs to implement a deep learning model tomorrow. But every business needs to be asking: What’s our plan for volatility?
If your current planning system can’t adapt quickly, AI isn’t a luxury. It’s a necessity.
Start small. Pilot a demand forecasting model. Layer machine learning into your inventory allocation. Explore tools that work with your current stack. The point isn’t to automate everything overnight. It’s to start building the muscle of forecasting, adjusting, and repeating—at speed and scale.
Final Thoughts: In a Volatile World, Learning Systems Win
Predicting the future is hard. Adapting to it shouldn’t be.
AI doesn’t eliminate uncertainty. But it helps you stay one step ahead of it — by learning, evolving, and reacting faster than legacy tools ever could.
And in today’s supply chain landscape, that kind of agility isn’t just a strategic advantage. It might be the only way to keep up.