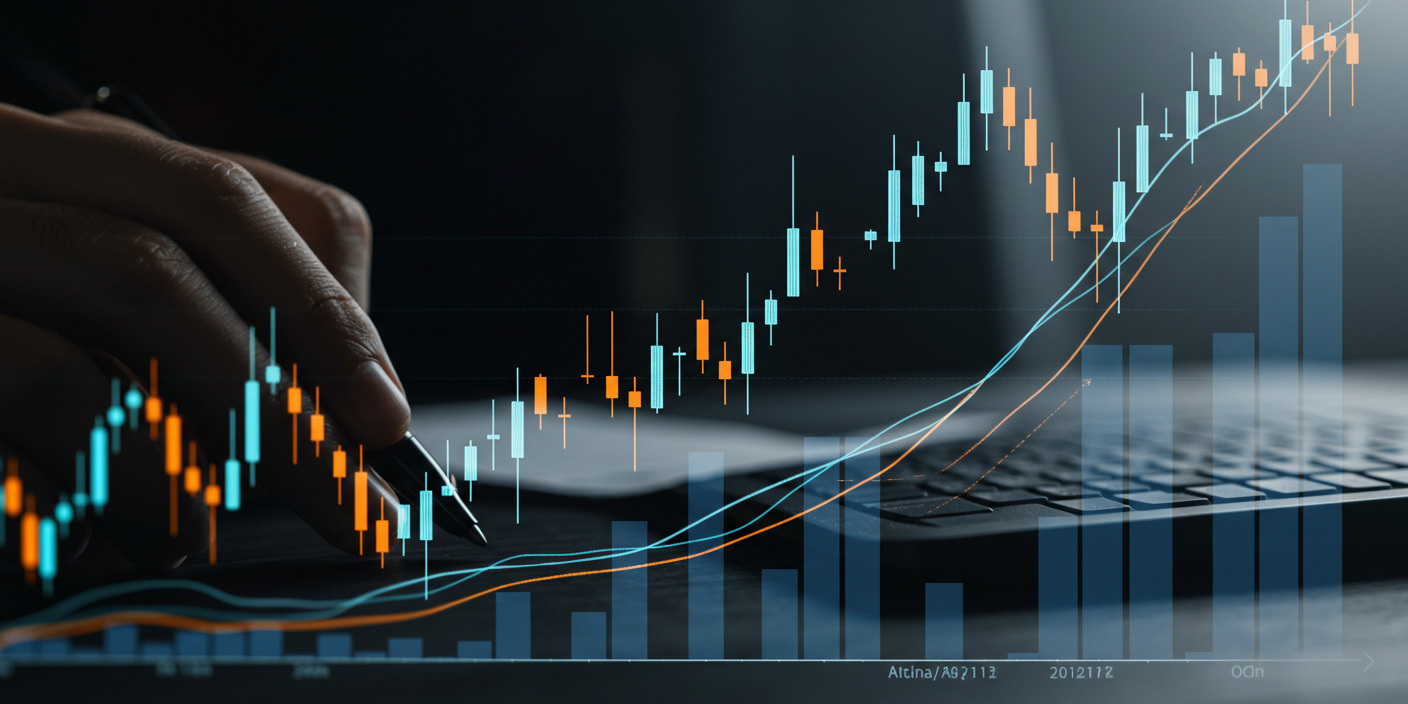
For decades, spreadsheets have been the backbone of financial forecasting. Rows, columns, macros — they’ve served CFOs, analysts, and planners well. But the world we operate in today doesn’t move at spreadsheet speed. Markets shift overnight. Supply chains fracture in a matter of days. Consumer behavior turns on a dime.
Spreadsheets weren’t built for that kind of volatility.
AI is. And early adopters are already finding that AI isn’t just another shiny tool — it’s a fundamentally different approach to seeing, predicting, and planning for what’s next.
Let’s dig into why AI is redefining financial forecasting, how it’s being used today, and what you need to watch out for as you cross into this new territory.
Why Traditional Financial Forecasting Is Cracking Under Pressure
Forecasting, traditionally, has been about historical pattern matching. We look at last year’s revenues, tweak for known variables like seasonality or market shifts, and extrapolate forward.
It works — until it doesn’t.
Problems with traditional models:
-
Linear assumptions: Many models assume stable growth or decline, which falls apart in chaotic environments.
-
Lagging indicators: By the time you notice something changing in the data, it’s often too late to pivot.
-
Human bias: We’re wired to overemphasize recent trends or underestimate unlikely events.
-
Limited variables: Spreadsheets can only handle so much complexity before they become unmanageable.
When COVID hit, when supply chains buckled, when interest rates jumped faster than anyone predicted — organizations relying solely on traditional forecasting tools struggled. Those experimenting with AI? They adapted faster.
How AI Changes the Game
AI doesn’t just run faster calculations. It changes how you think about forecasting altogether.
Here’s what AI brings to the table:
-
Pattern recognition at scale: Machine learning can find relationships between hundreds or thousands of variables — economic indicators, weather patterns, consumer sentiment — that a human analyst would never think to test.
-
Continuous learning: AI models don’t wait for quarterly reviews. They update predictions dynamically as new data flows in.
-
Scenario generation: Instead of one “best guess” forecast, AI can simulate hundreds of scenarios based on different assumptions and external shocks.
-
Anomaly detection: AI can flag when actual results start to drift from expected patterns much faster than manual reviews.
In short, you’re moving from forecast once and hope to forecast, monitor, adapt, repeat.
Real-World Examples: Who’s Doing This Well?
Companies that were early to AI forecasting are already seeing real benefits:
-
American Express uses machine learning models to predict customer churn and credit risk in near real-time, improving retention strategies and portfolio quality.
-
UPS deployed AI-based forecasting to optimize logistics during the pandemic, handling wildly fluctuating shipment volumes without collapsing under demand.
-
J.P. Morgan’s LOXM platform uses AI to forecast and execute trades optimally across volatile markets, reducing costs for institutional clients.
These aren’t theoretical pilots. They’re operational systems generating measurable improvements in resilience, cost control, and strategic agility.
What AI-Driven Forecasting Actually Looks Like
So what does this look like day-to-day inside a company?
-
Instead of quarterly reforecasts, you’re looking at models updating forecasts weekly — or even daily — as fresh data streams in.
-
Instead of “best-case/worst-case” assumptions crafted manually, your team runs thousands of Monte Carlo simulations at the click of a button.
-
Instead of one forecast based on a gut-feel top-line growth estimate, you have demand curves tied to real-time shifts in online sentiment, commodity prices, or regional economic health.
It’s not just faster. It’s smarter. It’s adaptive. And it gives finance teams space to move beyond number crunching and into true strategic advising.
Challenges and Limits You Shouldn’t Ignore
Of course, no technology is a free lunch. AI-driven forecasting brings its own set of challenges:
-
Data quality: If your operational, CRM, or ERP data is messy, AI will surface those flaws brutally.
-
Model explainability: Black-box forecasts that can’t be explained are a non-starter for CFOs, boards, and regulators.
-
Overfitting risks: AI can get too good at modeling noise if not tuned carefully, leading to false precision.
-
Ethical considerations: If financial models recommend actions that disadvantage vulnerable groups (e.g., raising lending standards during economic hardship), companies need frameworks to govern decision-making.
Good governance, transparent model validation, and hybrid human-AI workflows are crucial to avoid these traps.
Why Early Adopters Have an Advantage
If you’re thinking, “This sounds complicated,” you’re right. That’s exactly why early adopters have an edge.
While others are still stuck optimizing their spreadsheets, early movers are:
-
Building institutional muscle memory around continuous learning
-
Creating datasets that future-proof forecasting models
-
Training teams to partner with, not fear, AI recommendations
And when disruptions inevitably hit — and they will — it’s these companies that will adjust faster, invest smarter, and win bigger.
Final Thoughts: The Future Isn’t Linear
Spreadsheets built for steady growth don’t cut it anymore. Markets don’t move linearly. Neither should your forecasts.
AI-driven forecasting isn’t about predicting the future perfectly. It’s about equipping your organization to navigate uncertainty better than the competition.
It’s about trading in guesswork for insight. Slow reactions for rapid iteration. Static assumptions for living, breathing forecasts that evolve with the world around you.
And the companies that embrace that shift today? They’ll be the ones writing tomorrow’s success stories.
Maybe it’s time to stop wrestling with spreadsheets — and start forecasting like the future depends on it. Because it probably does.